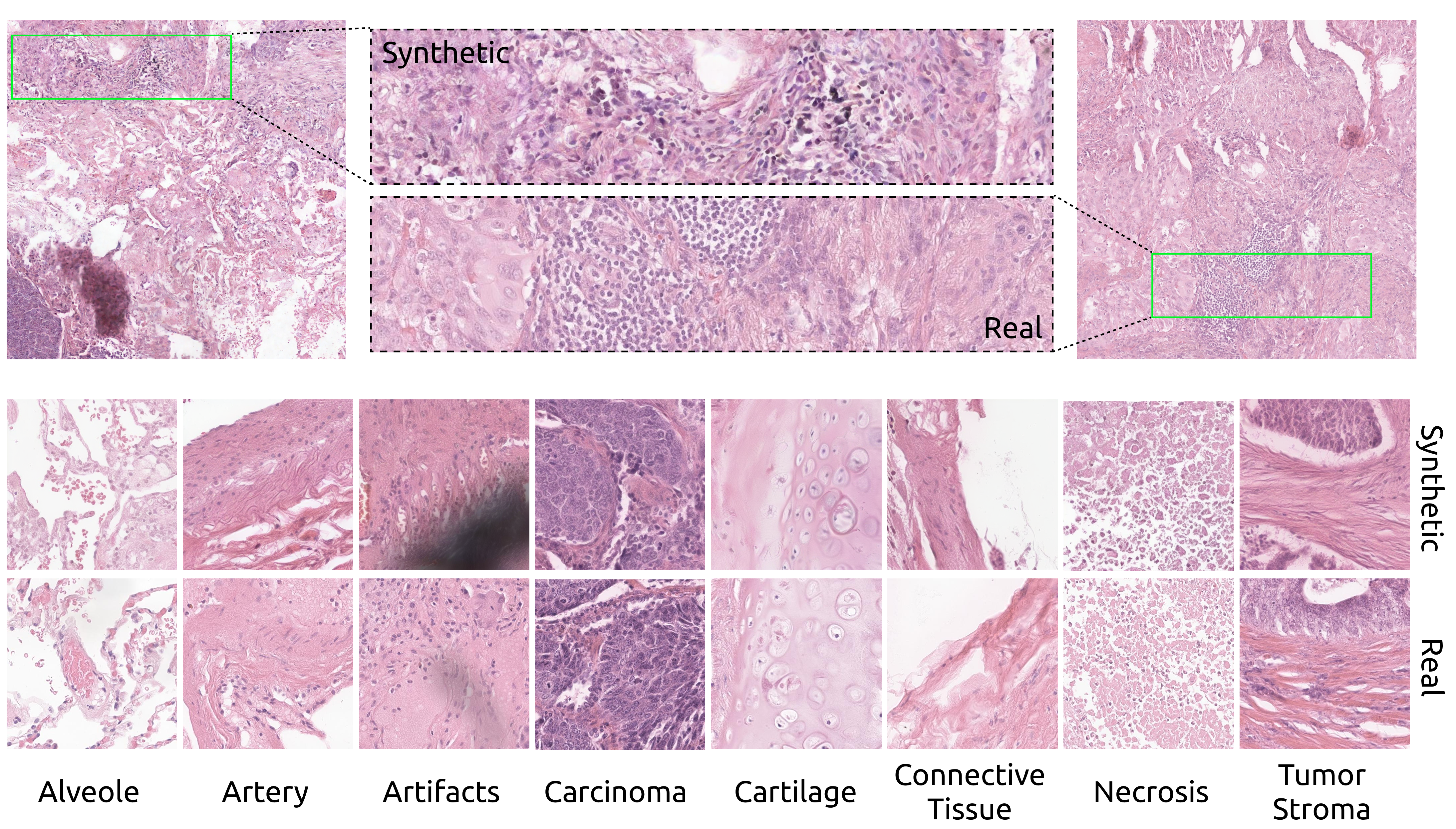
We present DiffInfinite, a hierarchical diffusion model that generates arbitrarily large histological images while preserving long-range correlation structural information. Our approach first generates synthetic segmentation masks, subsequently used as conditions for the high-fidelity generative diffusion process. The proposed sampling method can be scaled up to any desired image size while only requiring small patches for fast training. Moreover, it can be parallelized more efficiently than previous large-content generation methods while avoiding tiling artefacts. The training leverages classifier-free guidance to augment a small, sparsely annotated dataset with unlabelled data. Our method alleviates unique challenges in histopathological imaging practice: large-scale information, costly manual annotation, and protective data handling. The biological plausibility of DiffInfinite data is validated in a survey by ten experienced pathologists as well as a downstream segmentation task. Furthermore, the model scores strongly on anti-copying metrics which is beneficial for the protection of patient data.
Comparison between real and synthetic images (2048x2048 pixels)
DiffInfinite conditioning control visualization. The images is is generated from the squared mask shown (2048x2048 pixels). This output doesn't have biological meaning because the generated class depends on the mask shape and its neighbours. The mask generation is necessary to give this spatial information.
Generated images conditioned on the synthetic segmentation masks.
Unknown
Carcinoma
Necrosis
Tumor Stroma
Others
Image inpainted with different classes and different strengths of conditioning (small ω corresponding to less diversity)
@misc{aversa2023diffinfinite,
title={DiffInfinite: Large Mask-Image Synthesis via Parallel Random Patch Diffusion in Histopathology},
author={Marco Aversa and Gabriel Nobis and Miriam Hägele and Kai Standvoss and Mihaela Chirica and Roderick Murray-Smith and Ahmed Alaa and Lukas Ruff and Daniela Ivanova and Wojciech Samek and Frederick Klauschen and Bruno Sanguinetti and Luis Oala},
year={2023},
eprint={2306.13384},
archivePrefix={arXiv},
primaryClass={eess.IV}
}